- About
- Admissions
- Study at AUS
- Prospective Students
- Bachelor's Degrees
- Master's Degrees
- Doctoral Degrees
- Admission Publications
- International Students
- Contact Admissions
- Grants and Scholarships
- Sponsorship Liaison Services
- Testing Center
- New Student Guide
- File Completion
- New Student Orientation
- Payment Guide
- Executive Education
- Students with Disabilities
- Academics
- Life at AUS
- Research and Graduate Studies
- Contact Us
- Apply Now
- .
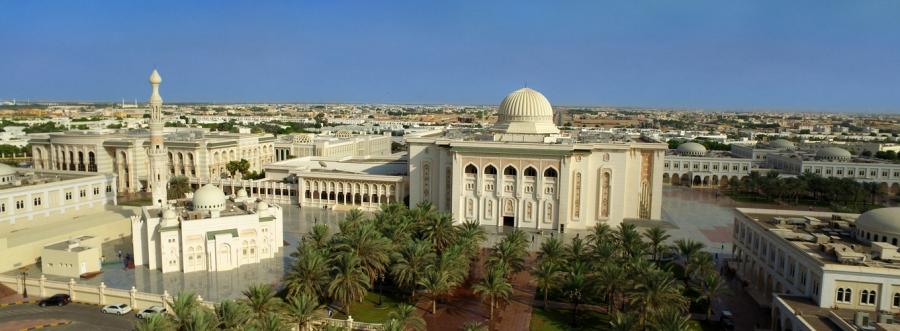
Detection of Traffic Incidents Using Machine Learning Techniques (April 2023)
PhD dissertation final oral defense presentation by PhD-ESM candidate Osama ElSahly
Traffic volumes on freeway systems usually grow because of the population and economic growth around the world. The significant increase in traffic volumes results in major delays on road networks, especially on freeway systems. The increase in delays led many countries to develop Traffic Management Centers (TMC) that include several functions to manage the traffic within a specific city/region. One of the vital functions in a TMC is the incident detection and management component. Traffic incidents are a major challenge faced by transportation systems worldwide, causing delays, air pollution, and significant economic losses. In response to this challenge, the development of efficient and reliable incident detection methods has become a critical research area. This dissertation proposes new machine learning models to detect traffic incidents on freeways, using supervised algorithms to classify traffic data collected from the freeway system. The study focuses on developing generic incident detection models that consider multiple variables simultaneously and studying their impact on incident detectability. To achieve this, the study used the well-known microsimulation software VISSIM to simulate various incident scenarios at different traffic volumes and locations. The proposed models, which include the Random Forest and Multilayer feedforward Artificial Neural Network, were trained and tested on simulated traffic data that represent both normal and incident conditions. The developed models achieved excellent performance, surpassing existing models in the literature, with the Artificial Neural Network model achieving a detection rate (DR) of 95.96%, a mean time to detect (MTTD) of 1.78 minutes, and a false alarm rate (FAR) of 1.01% during training, and a DR of 100%, MTTD of 2 1.6 minutes, and FAR of 1.29% during testing. Similarly, the Random Forest model achieved a DR of 96.97%, MTTD of 1.21 minutes, and FAR of 0.62% during training, and a DR of 100%, MTTD of 1.17 minutes, and FAR of 0.862% during testing. The proposed models can significantly improve traffic performance on freeways, especially during incidents, and benefit both local and international transportation agencies.
The study's contribution lies in developing efficient and reliable incident detection models that consider multiple variables simultaneously, improving traffic safety, reducing congestion, saving lives and properties, and reducing pollution.
PhD Supervisor: Dr. Akmal Abdelfatah, PhD-ESM Program Coordinator
For more information, contact [email protected].