- About
- Admissions
- Study at AUS
- Prospective Students
- Bachelor's Degrees
- Master's Degrees
- Doctoral Degrees
- Admission Publications
- International Students
- Contact Admissions
- Grants and Scholarships
- Sponsorship Liaison Services
- Testing Center
- New Student Guide
- File Completion
- New Student Orientation
- Payment Guide
- Executive Education
- Students with Disabilities
- Academics
- Life at AUS
- Research
- Contact Us
- Apply Now
- .
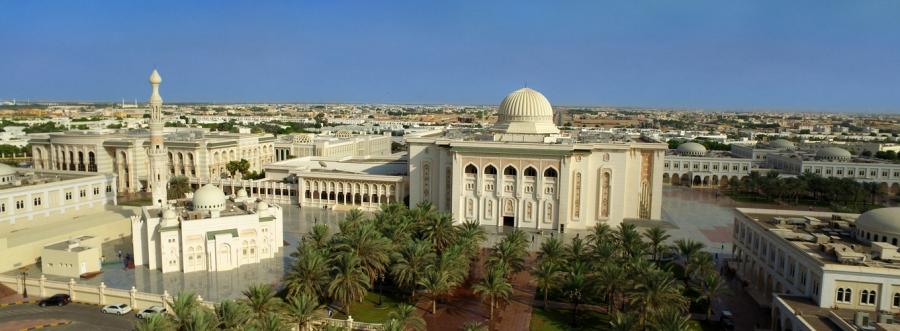
PhD Dissertation Final Oral Defense (April 2024)
Title of dissertation: A Machine Learning Model Based Schedule of Photovoltaic Solar Plant Dust Cleaning
Name of Candidate: Haneen Abuzaid | PhD in Engineering - Engineering Systems Management
Name of Supervisor: Dr. Mahmoud Awad
Abstract
Photovoltaic (PV) systems are widely utilized renewable energy resources, playing a vital role in sustainable energy generation worldwide. Nonetheless, their performance is significantly reduced by dust accumulation, emphasizing the importance of effective cleaning strategies. This research aims to enhance the overall performance of PV systems by improving maintenance practices, particularly focusing on the methods and schedules for PV cleaning. To achieve this, a comprehensive literature review spanning from 2010 to 2024 is conducted, concerning factors influencing PV performance, and current PV cleaning methods, with ongoing discussion and validation from PV experts, actively engaged in the PV field, to bridge theory and practice. The most influential factors include meteorological variables, PV specifications, system design, dust characteristics, sustainability considerations, and operational factors. Using a Multi-Criteria Decision-Making (MCDM) model, specifically the Analytic Network Process (ANP), the study proposes an optimal PV cleaning method, identifying partially automated cleaning as the most suitable for utility-scale PV projects in MENA. Additionally, the research offers a robust cleaning schedule by predicting the PV Performance Ratio (PR), a standardized metric that is widely used in performance-guaranteed contracts, utilizing machine learning algorithms. Two prediction models are proposed: time-series prediction models using LSTM, ARIMA, and SARIMAX algorithms for predicting the PR, and a threshold-based ensemble voting classifier using RF, Log and GBM for predicting the cleaning process, with three large and distinct datasets validation. Results indicate the efficacy of SARIMAX in PR prediction, with high R2 values and low errors across case studies. The ensemble voting classification model achieves satisfactory performance accuracy in predicting cleaning processes. Notably, PR predictive models outperform cleaning process classification models. Moreover, varying features’ importance outcomes across case studies highlight the necessity of including location-specific conditions for optimal PV cleaning strategy. The findings provide robust guidelines for PV system stakeholders, aiding informed decision-making and enhancing the sustainability of PV cleaning processes.
For more information, please contact [email protected].