- About
- Admissions
- Study at AUS
- Prospective Students
- Bachelor's Degrees
- Master's Degrees
- Doctoral Degrees
- Admission Publications
- International Students
- Contact Admissions
- Grants and Scholarships
- Sponsorship Liaison Services
- Testing Center
- New Student Guide
- File Completion
- New Student Orientation
- Payment Guide
- Executive Education
- Students with Disabilities
- Academics
- Life at AUS
- Research
- Contact Us
- Apply Now
- .
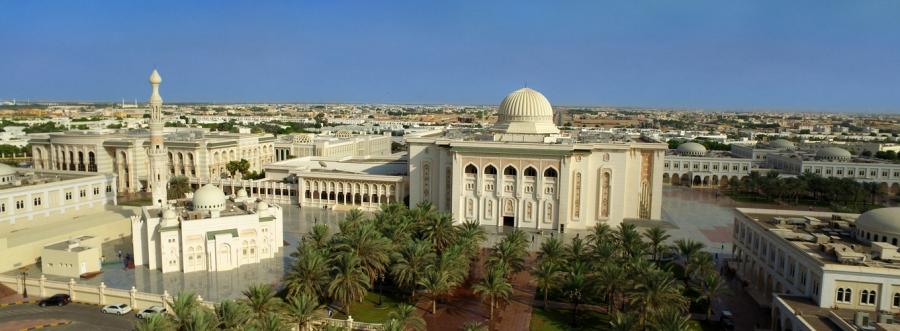
PhD Dissertation Final Oral Defense (April 2025)
Title of Dissertation: Utilization Of Machine Learning Techniques for Improving The Diagnosing Of Thyroid Disorders
Candidate: Areej Mohammed
Program: PhD in Engineering Systems Management
Supervisors: Dr. Hussam Alshraideh and Dr. Abdulrahim Shamayleh
Abstract :
Thyroid disorders are among the most common endocrine diseases, disrupting hormone levels and organ function. Timely and accurate diagnosis is critical to prevent severe health consequences. While machine learning has the potential to improve thyroid disorders diagnosis, current studies depend on invasive, costly, and time-consuming approaches. In this research, A holistic framework is presented to build a novel, user-friendly, and highly accurate risk calculator for diagnosing thyroid disorders, offering a significant advancement in non-invasive, cost-effective, and time-saving diagnostic tools. This innovative risk calculator achieves an impressive overall accuracy of 99.13% and attains a macro average precision of 91.28%, recall of 91.42, and F1-score of 91.34%, which highlights its exceptional diagnostic capability. The development of this comprehensive diagnostic tool was achieved through three main phases. In the first phase, population-based reference intervals were established using a robust control group identification approach, which was then used for accurate prevalence assessment of thyroid disorders. These reference intervals form the foundation for the risk calculator, serving as the initial layer of its framework. The second phase involved the development of an explainable hybrid machine learning model to predict TSH levels with an R² of 94.2% and an RMSE of 0.015, using fully non-invasive features, including demographics, family history, symptoms, and aggregated symptom scores. The model performance was then enhanced while reducing complexity by implementing advanced feature selection techniques. The third phase involved the integration of the first two phases' outcomes with four binary classification models to build the final risk calculator. The first model classifies individuals into normal versus non-normal thyroid status, followed by three subclassification models to distinguish hypothyroidism and hyperthyroidism and to differentiate between subclinical and overt cases. SHAP analysis complemented these models to enhance the interpretability of the proposed risk calculator. This research introduces a novel methodology that advances the diagnosis of thyroid disorders by accurately presenting a non-invasive diagnostic tool with wide applications for disease diagnosis and management.
For more information, please contact [email protected].