- About
- Admissions
- Study at AUS
- Prospective Students
- Bachelor's Degrees
- Master's Degrees
- Doctoral Degrees
- Admission Publications
- International Students
- Contact Admissions
- Grants and Scholarships
- Sponsorship Liaison Services
- Testing Center
- New Student Guide
- File Completion
- New Student Orientation
- Payment Guide
- Executive Education
- Students with Disabilities
- Academics
- Life at AUS
- Research
- Contact Us
- Apply Now
- .
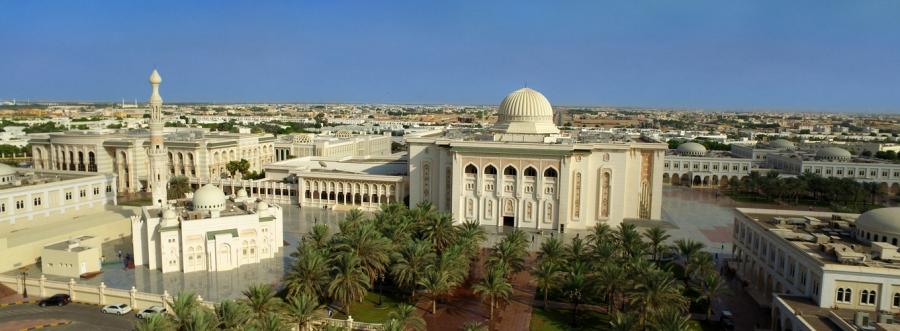
PhD Dissertation Final Oral Defense (May 2025)
Title of Dissertation: Optimal Participation of Virtual Power Plants in Energy Markets
Candidate: Fatemeh Marzbani
Program: PhD in Engineering Systems Management
Supervisors: Dr. Ahmed Osman
Abstract: The global transition toward cleaner energy systems has significantly accelerated the integration of renewable energy sources into modern power networks. This shift has driven the evolution from conventional power systems to smart grids. However, smart grids often lack the flexibility required to manage the variability and intermittency introduced by large-scale RES deployment. As a result, there is an increasing need for more advanced and adaptive energy management approaches. In this context, virtual power plants have emerged as a promising solution. By leveraging advanced communication and control technologies, virtual power plants enable the coordinated operation of distributed energy resources, thereby enhancing system flexibility, resilience and efficiency. Moreover, the growing participation of prosumers, the decentralization of energy generation, and the evolution of electricity markets toward liberalized models have reinforced the necessity for robust and intelligent virtual power plants-based energy management frameworks. This dissertation presents a hierarchical, data-driven decision making framework for the optimal operation, coordination and market participation of virtual power plants. The proposed framework comprises three interconnected stages. In the first stage, a super learner-based ensemble model is developed to improve forecasting accuracy for key VPP parameters, including load, renewable generation and electricity prices. This predictive layer effectively addresses multi-source uncertainty and strengthens the reliability of operational planning. The second stage introduces a feature-driven deep K-means clustering technique for forming virtual power plants dynamically based on both topological and operational characteristics. In the third stage, a two-level optimization approach is implemented, which consists of system-level scheduling and electricity trading. Two trading mechanisms are explored: a cost-minimizing bilateral model and a bonus-based market-oriented mechanism that incentivizes inter-virtual power plants collaboration. The framework is evaluated through a detailed case study using a modified IEEE 33-bus distribution system and real-world hourly data. The results indicate substantial improvements in forecasting accuracy, operational cost savings and enhanced coordination across virtual power plants. Additionally, the proposed trading strategies facilitate the use of surplus renewable energy, thereby reducing curtailment and minimizing grid imports. These outcomes contribute to both economic efficiency and emissions reduction. Overall, the findings demonstrate that the proposed framework is a practical and adaptable solution for intelligent energy management in decentralized and dynamic power systems.
For more information, please contact [email protected].